The purpose of this article is to suggest a simple and functional approach to cataloguing the risks arising from the implementation of artificial intelligence within organisations.
Preliminary Concepts
Over the last few years, cyber risks have evolved to such an extent that they have become a real element of concern and interest at international level. The need to catalogue them according to a specific taxonomy has resulted from this evolution, which, over time, has led some types of attack to disappear and others to assert themselves. Categories, sub-categories and specialisations were created to try to gather threats into a structure that was both useful and understandable.
The same applies to artificial intelligence, but with a higher complexity index: considering the implementation of artificial intelligence within an organisation as if it were a merely technical process would be a mistake. Certainly, implementing an algorithmic model is, predominantly, an engineering process, but there are also strong implications in the legal world. An algorithmic model is, in fact, developed by a supplier and sold to a customer, not much different from any software. However, whereas normal software offers static and predictable results, an artificial intelligence algorithm has a dynamic behaviour that we could call, in some respects, plastic because it changes over time, as the conditions of use and query change. It is therefore a unique case in the history of computer science and must be treated as such.
Classification by phase
Phase classification aims to place the threat (whether accidental or deliberate) within the three main phases of the life of an artificial intelligence model.

The three phases are:
- TRAINING: understood as the phase in which the provider prepares the artificial intelligence model for the purposes for which it was designed and built. This phase is normally the responsibility of the supplier and returns an almost complete algorithmic model.
- REFINING: understood as the fine-tuning phase of the trained model. This phase, also called ‘fine-tuning’, is a process in which a pre-trained model is further trained on organisation-specific data to better adapt it to the user’s needs. This phase is common when one wants to improve the quality of the model’s responses in a particular context, correct any bias or adapt it to a specific domain. Normally this phase is carried out in a controlled usage context, and can be performed either at the supplier’s premises or on the customer’s systems (if they have the appropriate infrastructure to support it).
- USE: understood as the phase of use of the model by the user, with monitoring of the responses provided by the model and any corrections by the supplier.
The phases, in this case, also suggest a primary and a secondary responsibility.
Phase | Primary Responsibility | Secondary responsibility |
---|---|---|
Training | Supplier | – |
Refinement | Supplier | Customer |
Use | Customer | Supplier |
As can be seen, there is a literal and understandable transition of primary responsibility from the supplier to the customer that should also be reflected within the contracts concluded between the parties. This transition is physiological and quite obvious: the object of supply (the model) is handed over for use by the supplier to the customer, who consequently becomes primarily responsible for it. But it is equally clear that contracts governing the design, implementation and use of artificial intelligence will have to take these aspects into account. As an example, in the training phase, the algorithmic model may be exposed to very specific attacks, including poisoning attacks:
- Targeted Poisoning: they result in a violation of the integrity of the model by altering its prediction on a small number of targeted samples.
- Backdoor Poisoning: similarly to targeted poisoning attacks, targeted poisoning leads to a violation of the model’s integrity, but in this case the aim is to mislead the model in response to a specific data sample (called a trigger).
The training phase, in fact, allows the attacker to access the model structure and training data in a direct manner and with far more invasive results.
Data protection aspects
The type of attack suggests an important aspect, namely how the provider intends to protect the model data. In fact, if the model is not adequately protected at a later stage, specific attacks and offensive techniques could reach the training data. The security imposed by the supplier must therefore be of a high level, and the algorithmic model must necessarily be robust and guarantee protection towards the architecture, procedures, configuration and training data. This opens up a reflection on the role of contracts.
It will be necessary to draw up appropriate contracts in order to precisely establish the responsibilities pertaining to the supplier and the customer, based on specific aspects of their responsibility. For example: the customer is not the manager of the training data but uses it during its use, and if an attack on its infrastructure succeeds in reaching the algorithmic model by modifying it, it is not necessarily its overall responsibility for the lack of computer security. It will be necessary to distinguish the shortcomings that allowed the data breach from those that allowed the model to be changed, as the provider should have foreseen a series of measures to prevent such changes. Attacks such as the Membership Inference Attack, the Data Extraction Attack or the Model Inversion Attack, may exploit vulnerabilities in the model, which may be unknown to the user. As a result, these attacks could alter the model’s calculation system, resulting in damage to the end customer.
The importance of contracts and the responsibility of suppliers
Disciplining this complicated set of balances is the task of the contract and this raises the question of the importance of having competent professionals in the field, capable of regulating complex situations. The scenario becomes even more complicated when the model acquires, as input, data from other systems.
In that case, one would be led to believe that the handling of the alteration concerns the supplier of the input data, but not necessarily. The disturbance may not be in the ‘modification’ of the input data, but in the addition of an illicit value to the calculation model, which, as a consequence, will produce an error in the final result. In essence, along with the expected data, an unforeseen value could be inoculated that induces error in the algorithm. In this case, too, one would have to assess how the algorithm provider has protected the data input procedures, also in order to avoid so-called evasion attacks.
Responsibility in the model, system and ecosystem
ISO 27005 (including version 2022), differentiates risk according to system and ecosystem. This differentiation takes on particular relevance when one drops it into the discourse of artificial intelligence.
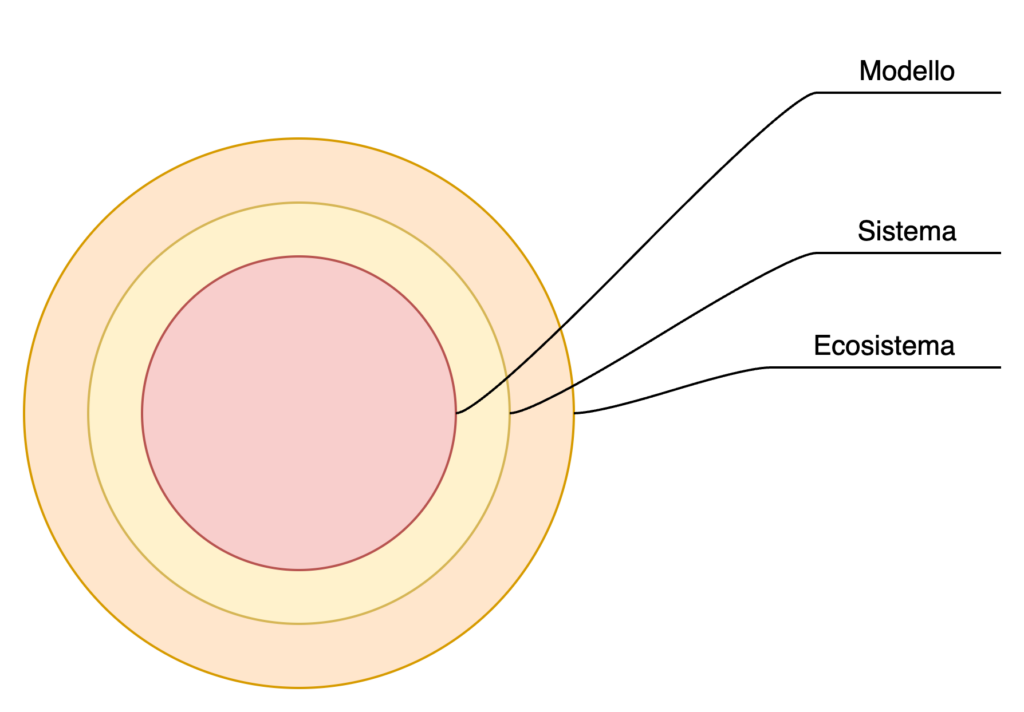
The algorithmic model, as we explained earlier, must have specific security measures to protect data and processing. These security measures, mostly imposed by the provider, are intended to protect it both in the training phase and in the subsequent phases of refinement and use by end users.
The system can be understood as the infrastructure required for the model to function. It is not limited to a single computer, or a single part of the infrastructure, but to all the resources necessary for the model to function and deliver the performance for which it was designed and built. This places the system in a more complex risk management dimension: one must, in fact, take into account all the aspects normally included in risk assessment, including: personnel, infrastructure, equipment, information, procedures, suppliers, databases, etc.
The ecosystem is the context in which the system operates and with which it regularly exchanges information. The ecosystem does not necessarily exist only in the utilisation phase: a model that interrogates external databases in the training phase is itself already connected to an ecosystem. The ecosystem presents other difficulties, the greatest of which, as is well known, is the impossibility of operating directly on the systems that make it up. The ecosystem is, by definition, dynamic and requires adaptive and ‘plastic’ cybersecurity approaches. The ecosystem can pose a threat to both the system and the model.
Risk dimensioning therefore becomes particularly relevant, especially when the model may be exposed to both system- and ecosystem-related risks.
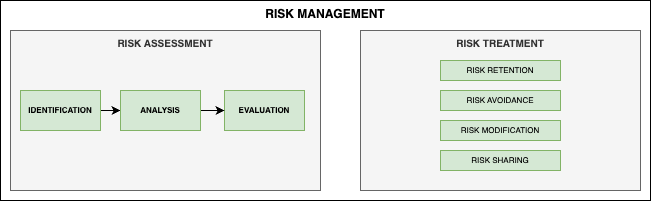
In the summary representation above, one can see the two essential moments of risk management: risk assessment and risk treatment. Both must take into account the three levels under consideration: model, system and ecosystem.
A possible subdivision
In the article‘Risk Management and Artificial Intelligence‘, the main types of attacks were addressed. On the basis of those typologies, in a highly simplified manner, an attempt was made to attribute responsibility for cybersecurity to one of two parties.
- The supplier of the model is the one who produces the algorithmic model and sells it.
- The purchasing company buys it in order to be able to give it for use by its staff or users.
Type of attachment | Model Supplier | Buyer Company |
---|---|---|
Evasion Attacks | X | |
Availability Poisoning | X | X |
Targeted Poisoning | X | X |
Backdoor Poisoning | X | |
Model Poisoning | X | X |
Data Reconstruction | X | X |
Membership Inference | X | X |
Model Extraction | X | |
Property Inference | X |
The table essentially shows two situations: there are cases where liability is clearly attributable to one of the two actors involved (see the case of Model Extraction). On the other hand, there are cases of co-responsibility that have to be regulated as stated so far.
Conclusions
The contract maintains its central role in regulating roles, responsibilities, management and communication methods. In this sense, it is good to remember how much this is provided for by standards such as ISO 27001 or the CSCs that, although they do not use the word‘contract‘ but‘agreement‘, impose the formalisation of these aspects. For example, in the case of ISO 27001:2022, paragraph 4.2 states that:
4.2 Understanding the needs and expectations of interested parties
The organisation shall determine:
(a) interested parties that are relevant to the information security management system;
b) the relevant requirements of these interested parties;
c) which of these requirements will be addressed through the information security management system.
The requirements of interested parties can include legal and regulatory requirements and contractual.
The challenge will therefore be to have a clear but also well-defined contractual framework with appropriate allocation of responsibilities, procedures and possible penalties. This, however, will also require refined technical skills in order to understand without any doubt the nature, origin and details of incidents. In essence, it is essential to have certainty of what happened in order to make the contract governing the relationship between the parties enforceable.