The purpose of this article is to show the evolution of ISO 27005, to present a compatible and alternative methodological paradigm for cyber risk management, and to observe some of the threats concerning artificial intelligence.
ISO 27005:2018 and general considerations
ISO 27005 of 2018 (the third edition), presents a very interesting table in Annex-C that includes the origin of the most common threats, the motivations behind the attacks and the possible consequences. The table, while important, is affected by the passage of time and deserves some reflection; the 2018 ISO includes 5 types of subjects:
- Hacker
- Cybercriminals
- Terrorists
- Industrial espionage
- Internal (meaning internal members of an organisation)
At first glance, the proposed subjects are quite distinct, and each of them has been attributed motives for attack; for example, cybercriminals have been attributed the following:
- Computer criminalsPolylang
placeholder do not modify
These reasons, in turn, can give rise to various consequences that ISO proposes in further tables and lists. In the writer’s opinion, the essential problem with ISO 27005:2018 is that it is no longer able to adhere to the current risk scenario. Let us take an example: consider the motivation related to “blackmail”, the ISO associates this motivation with the following subjects
- BlackmailPolylang
placeholder do not modify
It is well known, however, that recent cyber attacks are based on the logic of blackmail, whenever the victim refuses to pay the ransom demanded by hackers. By now, this is a well-known and established practice: taking into account the threat made by ransomware, the strategy of double extortion is based on blackmail. The Treccani Encyclopaedia defines blackmail:
Extortion of money, or other illicit gains, by threats constituting moral coercion
Consequently, it would be more appropriate to associate the motivation blackmail with both the terrorist threat and the hacker threat and also, consequently, the cybercriminal threat.
ISO 27005:2022 and general considerations
ISO 27005:2022 radically changes the risk management paradigm: the standard proposes a‘risk source‘ approach and a greater focus on the organisation’s operational scenarios. An example of this is the following scheme called‘Risk assessment based on risk scenarios‘.
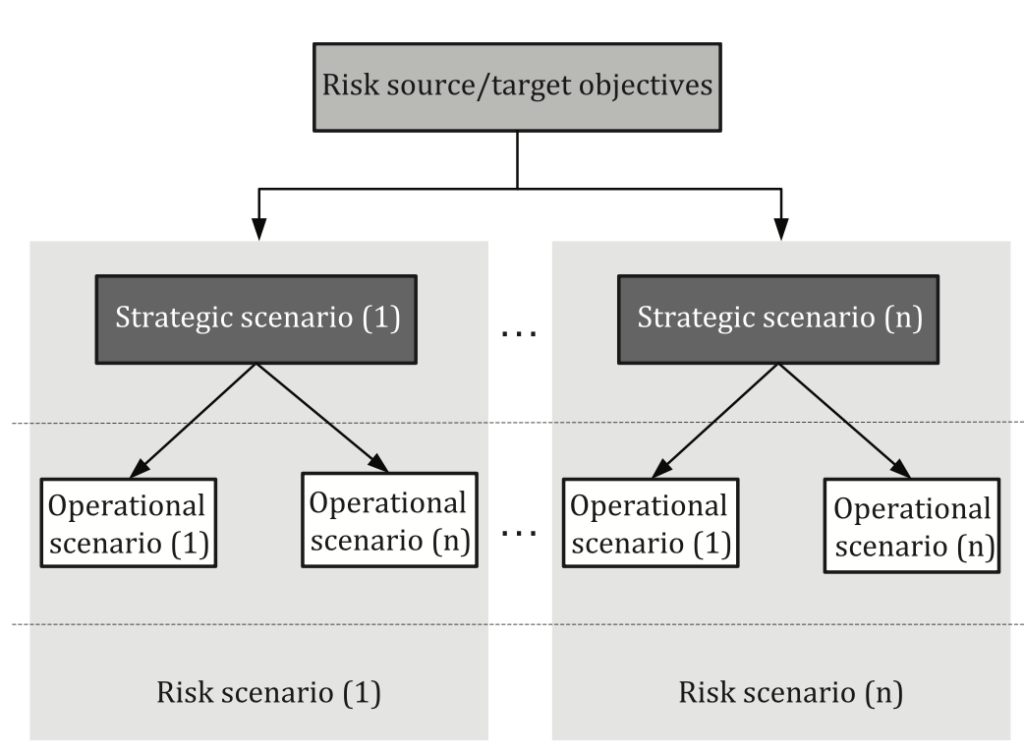
The evolved approach takes into account the fact that operational scenarios may be of varying importance under varying time conditions: a ten-year project will retain its strategic value over a period of time not necessarily equal to the entire project length. This requires that all factors that may be impacted by the threat be taken into account. In this sense, it is useful to note that ISO 27005:2002 presents a special scheme for event-based risk management.
Risk source | Description |
---|---|
State-related | States, intelligence agencies |
Organised crime | Cybercriminal organisations (mafia, gangs, criminal outfits) |
Terrorist | Cyber-terrorists, cyber-militias |
Ideological activist | Cyber-hacktivists, interest groups, sects |
Specialised outfits | “Cyber-mercenary” profile with IT capacities that are generally high from a technical stand-point. |
Amateur | Profile of the script-kiddies hacker or who has good IT knowledge; motivated by the quest for social recognition, fun, challenge. |
Avenger | The motivations of this attacker profile are guided by a spirit of acute vengeance or a feeling of injustice. |
Pathological attacker | The motivations of this attacker profile are of a pathological or opportunistic nature and are sometimes guided by the motive for a gain. |
It is surprising to note how much the list of risk sources has evolved, expanded and become more detailed. In particular, it should be made clear that there can be a strong interaction between some of these categories: an example would be state-related and specialised outfits, which, in this period, are at the basis of some Russian cyber attacks against European targets. Cyber attacks perpetrated by a state(state-related), in fact, often make use of hired mercenaries(specialised outfits) to conduct the offensive. It is equally interesting to analyse the targeted targets listed in table A.9 of the standard and summarised here:
- SpyingPolylang
placeholder do not modify
- Strategic pre-positioningPolylang
placeholder do not modify
- InfluenzaPolylang
placeholder do not modify
- Obstacle to operationPolylang
placeholder do not modify
- Lucrative purposePolylang
placeholder do not modify
RoA model proposal
Very often, companies wonder how to approach the methodological models proposed by standards. When thinking about an asset, often the physical and not the logical ones are considered, or relevant evaluation aspects are overlooked. When in doubt about‘what‘ to include in the attack scenario, it is good to remember that there is a simple but effective method to avoid making mistakes. One must ask the question of thereason for attack. What reason would an attacker have for conducting an offensive? In attempting to answer this question, we will highlight both the assets involved, the consequences of the attack, and the threats that could impact the organisation.
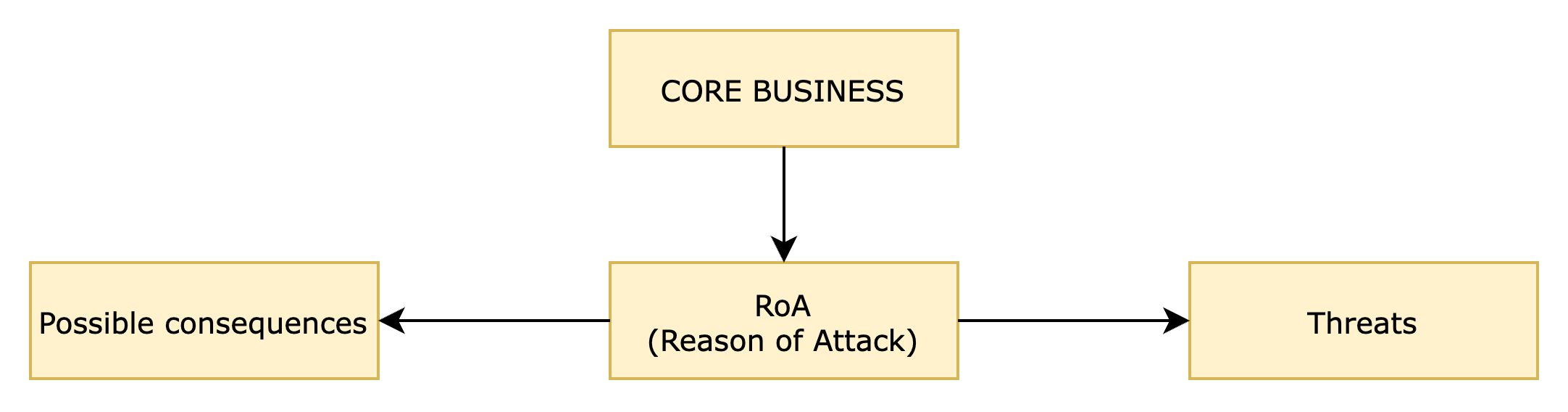
As can be seen from the diagram, the analysis must begin with the core business, which must be clearly identified. Being the core of the organisational activity, it is represented by a heterogeneous set of potential assets under attack; these could be infrastructure, documents, human resources. Each could be the basis of a Reason of Attack (RoA).
Since RoA can vary depending on the assets identified, this opens the scenario to hybrid attacks based on a multitude of techniques and ‘phases’: each asset can therefore be attacked with different methods, times and techniques and for this reason must be protected in as customised a manner as possible.
RoA, if properly analysed, can provide preliminary information on threats and possible consequences; the data obtained can be used to optimise defence and information protection strategies.
With regard to threats, it should be made clear that, although very relevant to identify, this methodological approach allows a greater tolerance for error in their detection. It is assumed that threats may change in type and ‘form’ as time passes and scenarios arise. Therefore, although relevant, the model takes into account their high variability and focuses the main attention on RoA and possible consequences.
Managing organisational complexity
The model takes into account the company’s core business and therefore a reduced set of information, processes and policies compared to the organisation’s total assets. This choice is deliberate in order to keep the model applicable, efficient, agile and manageable.
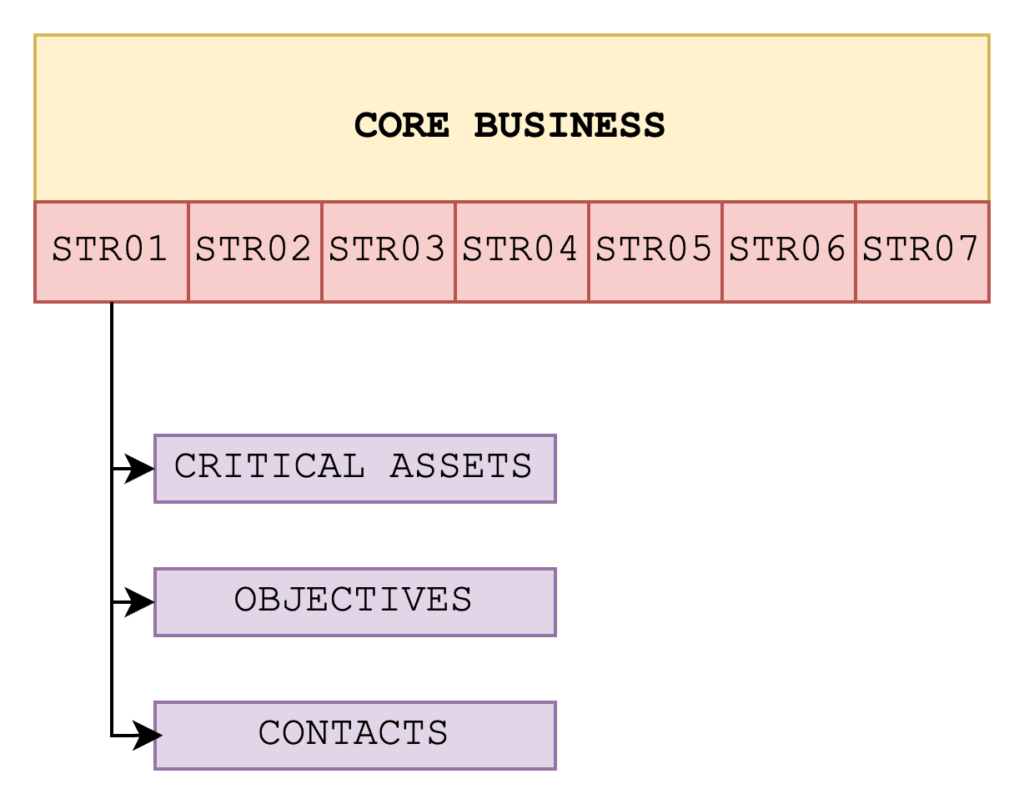
This assumes that the core business is composed of a series of strategies that are essential to the life of the organisation; in the case of the diagram, for example, there are seven. Each strategy constitutes a fundamental element without which the organisation could go under; they could be prototype production lines or services essential to the organisation. Each strategy is structured into critical assets, objectives and contacts.
- Critical assets are those that are essential to proceed with the development of the strategy and include devices, equipment, information, documents, data, without which it is impossible to proceed with the strategy. Critical assets do not include all those components that can be replaced, re-purchased, replaced without problems.
- Targets are the goal the strategy must reach. Generally, they could be an end product, an established service, a production level, a supply line target. Targets must be defended against attacks so that they can always be reached and so that the production line is not interrupted.
- Contacts, on the other hand, are the internal and external reference points useful for the maintenance of operations and the success of the strategy. This implies, for example, that a particular supplier affected by the strategy is considered a contact and must be monitored and managed as such. Standards such as ISO 27001, Critical Security Controls and regulations such as NIS 2, require the management of suppliers both prior to engagement, during supply and even at the close of the relationship.
For more information, please refer to the following articles:
Control and Management of Service Providers
NIS 2 – Information, Security and Suppliers
Regulatory Integration
RoA allows interfacing with current regulations such as European Directive 2555/2022 (NIS2), European Regulation 2554/2022 (DORA), ISO 27001, ISO27005, Critical Security Controls, European Data Protection Regulation (GDPR).
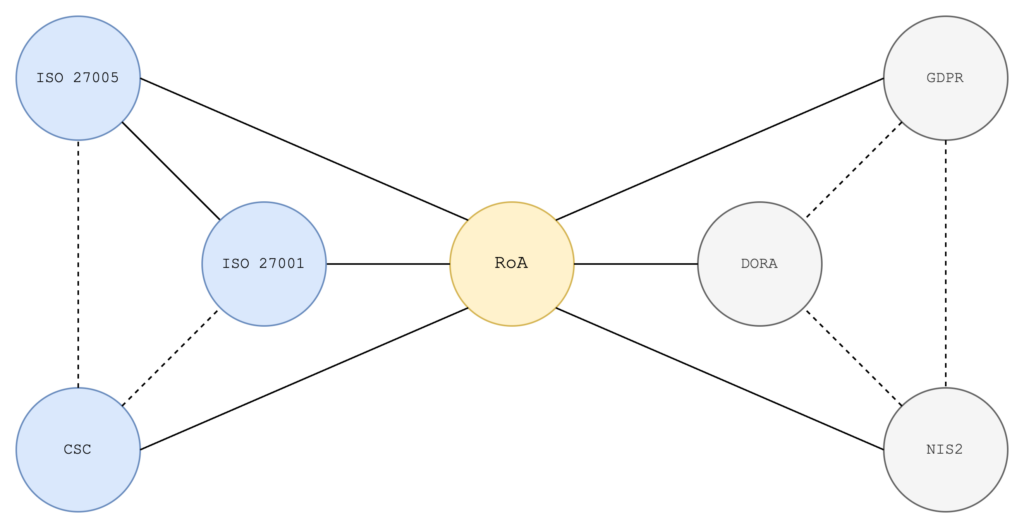
In the proposed model, RoA allows the organisation to focus on the main aspects of the strategy by capturing the most relevant objectives from the individual standards. RoA also responds to the principles of current regulations such as the GDPR. One thinks of the impact assessment (referred to in Article 35 of the regulation) or the principle of accountability: in these cases it is necessary to ask the question as to what to protect but above all from what and how, thus justifying a reason for attack.
Evolution from artificial intelligence
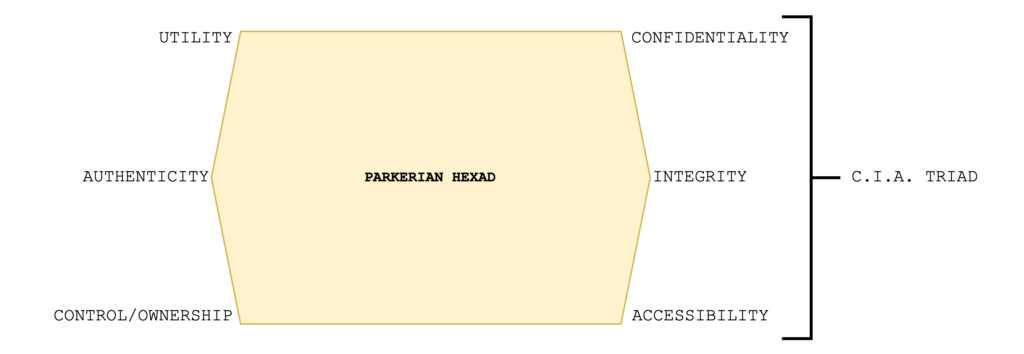
The RoA takes into account the increased complexity of the scenario, as anticipated in 1998 by Professor Donn B. Parker. With a larger volume of data, the attacker will have to ‘select’ those of his interest, trying to obtain this selection from an artificial intelligence system (integrated or third-party). An example could be a search engine equipped with an intelligent algorithm that allows queries on a thematic basis with respect to content. This would allow the attacker to select and prioritise files to be transferred and/or destroyed.
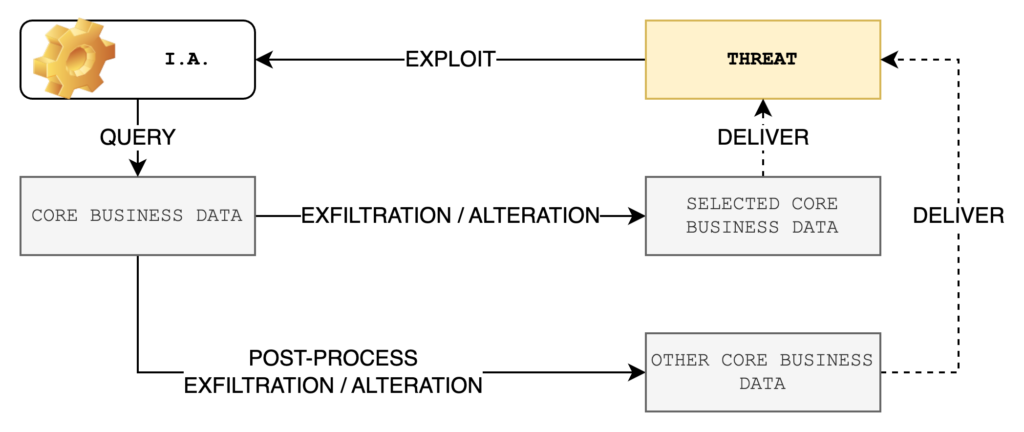
From a defensive point of view, it becomes complex to protect a large amount of data and, consequently, it will be necessary to identify those elements that are a priority for the core business and guarantee them maximum security. This does not mean that other elements will be outside the security perimeter, but that those belonging to the core business will be susceptible to greater vigilance. The paradigm applied is the same as in NIS 2 when it comes to core and important subjects: there is no difference between security measures but between frequency and intensity of vigilance.
Artificial intelligence and cyber risks
On 14 February 2025, the Agency for Digital Italy (AgID) published a document called‘Draft Guidelines for the Adoption of AI in Public Administration‘(link to document). Inside, many procedures for implementing artificial intelligence in public administration are described, as well as many techniques for protecting against risks arising from such implementation. In this regard, AgID clarifies that:
the PA MAY refer to the technical standards UNI ISO 31000 and ISO/IEC 23894
UNI ISO 31000 deals with risk management, while ISO/IEC 23894 deals with the explicit risks of A.I. and is entitled‘Information technology – Artificial intelligence – Guidance on risk management‘.
Attack techniques
AgID selects four main types of attack:
- Evasion Attacks: This category of attacks aims to generate an error in the model’s classification by introducing perturbations (often imperceptible to humans) in the model’s inputs, called adversarial examples. The aim is to induce the model to predict a value desired by the attacker or lead to a reduction in the model’s accuracy.
- Poisoning Attacks: a category of attacks aimed at degrading the performance of a model or making it generate a specific result by altering (poisoning) the model’s training data. It is divided into four subcategories
:Polylang placeholder do not modify
- Privacy Attacks: aims to compromise user information by reconstructing it from training data
.Polylang placeholder do not modify
- Abuse Attacks: they aim to alter the behaviour of a generative AI system to suit their own purposes, such as perpetrating fraud, distributing malware and manipulating information.
It should be borne in mind that these modes of attack, especially at a certain level of complexity, can be ‘mixed’ to give rise to a complex offensive action that is difficult to mitigate; let us analyse some examples among those suggested by AgID.
Example of Target Poisoning
Let us imagine a facial recognition system used for access control in a company. The AI has been trained on a dataset of authorised and unauthorised faces.
Phase 1: Injection of poisoned data
An attacker with access to the training dataset introduces modified images of himself, in which he appears similar to an authorised employee. This can be done in several ways:
- Adversarial Perturbation: small, imperceptible changes to pixels that deceive the model.
- Direct Data Poisoning: loading into the dataset false images of itself labelled as ‘authorised’.
Phase 2: Effects of the attack
After training, the system learns that the attacker is an authorised employee. He can now gain free access, while the system continues to function normally for all other users.
Why it is dangerous
- It is a difficult attack to detect: the behaviour of the AI is normal in most cases.
- The attack is aimed at a specific condition.
- If the attacker uses advanced techniques such as clean-label poisoning (without obviously incorrect data), the attack is almost invisible.
This technique can be applied in many areas, such as spam filtering, recommendation systems and fraud prediction models.
Example of Backdoor Poisoning
Imagine an AI-based traffic sign recognition system used in self-driving vehicles to identify stop signs and speed limits.
Phase 1: Manipulation of training data
An attacker introduces ‘poisoned’ images into the dataset, such as:
- Pictures of STOP signs with a small yellow sticker (the trigger).
- These images are incorrectly labelled as ‘100 km/h speed limit’ instead of ‘STOP’.
Since the number of modified images is small compared to the entire dataset, the model still functions correctly in most cases.
Step 2: Activation of the backdoor
After training, the model recognises STOP signs normally, except when there is a trigger (the yellow sticker). If an attacker applies this sticker to a real STOP sign, the car will not stop, believing it sees a ‘100 km/h’ sign.
Why is it dangerous?
- Hard to detect: the model seems to behave normally in standard tests.
- Selective activation: the backdoor is only activated by the specific trigger.
- Wide applicability: can be used in biometric authentication systems, content filtering, voice recognition, etc.
This technique has been demonstrated in various academic studies, such as the BadNets1 attack, and may have serious implications for AI security.
Practical example of Membership Inference Attack
Imagine a clinic that has trained an AI model to predict the probability of developing a rare disease based on medical data from patients.
Step 1: The predictive model
The clinic uses health data from thousands of patients to train a machine learning model. This model is then made available online as a public API, where users can enter their own data and obtain a prediction of disease risk.
Phase 2: The inference attack
An attacker wants to find out whether a specific person (e.g. a famous politician) was included in the training dataset. To do this:
- It obtains some public or approximate medical data of the victim.
- It queries the online model with similar data, observing the response.
- Compare the confidence of predictions: models tend to have more confidence in data seen during training than new ones.
If the model returns a prediction with high confidence for the victim data, it is likely that these were in the training dataset.
Why is it dangerous?
- Privacy violated: if the dataset contained sensitive information (e.g. medical records), an attacker could find out who was part of it.
- Wide applicability: similar attacks can be performed on facial recognition models, recommendations, credit analysis, etc.
- Itdoes not require access to internal data: it can only be executed by querying a public API.
This vulnerability has been studied in various contexts, and mitigations such as DifferentialPrivacy2 can reduce the risk: in 2020, the United States used DP to anonymise demographic census data.
Conclusions
The increase in information processing complexity has generated new attack modes and new offensive scenarios. In this sense, it is obvious and necessary for organisations to protect themselves, but if the methodology is wrong, the line of defence risks becoming too rigid and blocking. It is necessary to adopt one or more methodological models that are on the one hand adherent to standards, and on the other hand truly sustainable for organisations. All this, of course, is further complicated by the introduction of artificial intelligence, which requires specific controls and very close cooperation with suppliers.
Notes
- A BadNets attack is a form of backdoor attack applied to machine learning (ML) models, in particular Deep Neural Networks (DNN). This type of attack is carried out by intentionally introducing a backdoor (a sort of ‘Trojan horse’) during the model’s training phase, with the aim of making it work normally in most cases, but responding maliciously when it encounters a specific trigger. The mitigation of this type of attack is complex and is mainly based on the verification of data sources. ↩︎
- Differential Privacy (DP) is a technique used to protect the privacy of personal data in datasets analysed by machine learning algorithms, statistics or other computational processes. The main objective is to ensure that the results of the analysis do not reveal specific information about any individual, even if an attacker had access to the entire dataset. The advantages are the protection of information even with access to the data and the fact that it cannot be combined with other machine learning techniques for a more secure analysis. The disadvantages are a reduction in the precision of analysis, a difficulty of implementation in certain real-life contexts, and in any case it forces a compromise between privacy and usefulness of the data. ↩︎