Staying close to artificial intelligence, it is necessary to address a topic dear to jurists and humanists alike: the principle of discrimination applied to technology and, specifically, to algorithms.
What is discrimination
The term discrimination means:‘distinction, diversification or differentiation, made between persons, things, cases or situations‘ (Source: Treccani).
It is clearly a process that, to be correctly evaluated, requires to be placed in the right operational context; to make an algorithmic distinction means to expect an algorithm to behave differently depending on people, things, cases or situations. In some contexts, discrimination may be required, operated voluntarily, even sought after: think, for instance, of statistical contexts in which calculations may differ due to predetermined, known and voluntary conditions.
Yet the term discrimination also suffers from the eventuality that such diversification is used as a reprehensible process of penalisation. It is this case in particular that is examined by this article: the eventuality in which discrimination results in damage to third parties through culpable or malicious causes.
Malicious discrimination
The most obvious form of malicious discrimination is racism: a process of separation of rights and freedoms dictated by the racial origin of the individual and not supported by genuinely tenable motives. Malicious discrimination is essentially based on mainly human reasons such as fear and profit.
Profit means an advantage that can be economic but also social, political, technical, labour. It means a position of dominance, obtained at the expense of a minority that is deprived of something it is entitled to for no real reason.
Fear is that perfect propeller for behaviours and activities that we find in terms such as xenophobia, racism, anti-Semitism, homophobia, i.e. in behaviours of exclusion and penalisation of all that is not deemed fit for acceptance. To engage in malicious algorithmic discrimination is to deliberately train the algorithm to penalise a minority of the statistical universe under consideration.
Culpable discrimination
There is, however, a type of unintentional discrimination that we could call culpable discrimination and which generates phenomena similar to culpable discrimination but, precisely, unintentional and unsought. The reasons behind culpable discrimination are the same: faulty training in data and/or process and thus repeated diversification of the statistical reference universe according to unsearched criteria. In 2022, Il Sole 24 Ore devoted an article to algorithms that penalised women. Silvia Pagliuca’s article clearly states:
Amazon’s automated recruitment platform is programmed to sift through thousands of applications in a matter of seconds. However, these algorithms forfeit deep gender biases: since most of Amazon’s past hires are male, the algorithm tends to penalise CVs that include the word ‘woman’.
Training and the problem of sources
The algorithmic training phase is crucial. Whether it occurs through negligence or malicious causes, training risks being the weak point in the entire artificial intelligence chain. In the same article, Silvia Pagliuca provides a useful explanation of discriminatory causes:
This happens both because they learn from time series and because they may have been set with biased data that is not fully representative.
This explanation brings us back to the main problem of artificial intelligence (and not only): the reliability of sources and their understanding. At present, when you ask an algorithm to mention the sources used as the basis of the algorithmic calculation, you get a negative answer: no satisfactory information is provided.
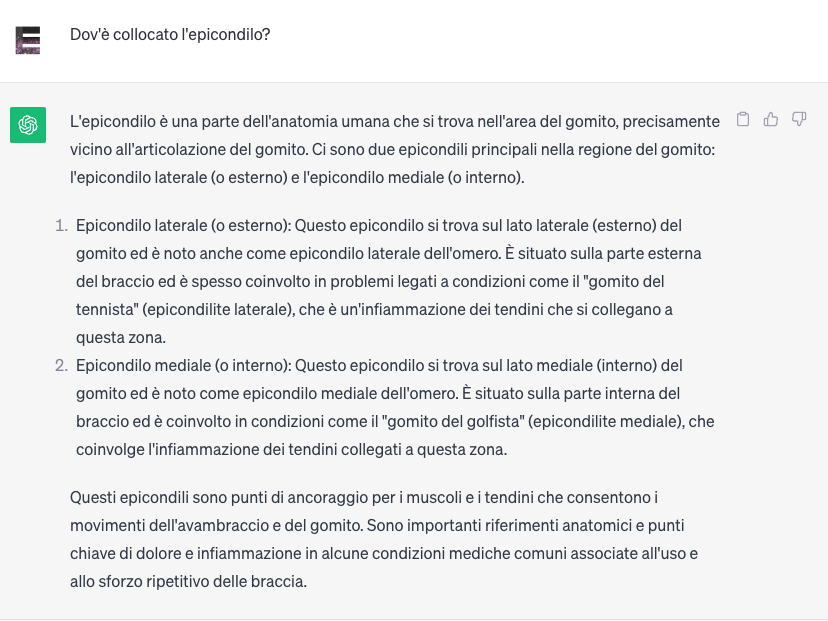
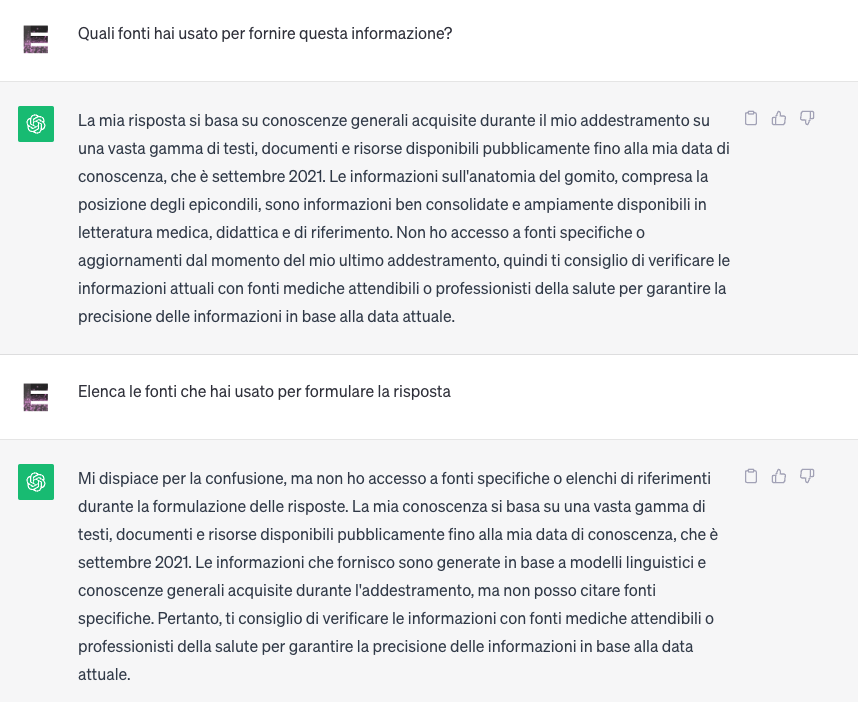
Today, the internet suffers from an incredible lack of reliability, we could say that everything is a source and nothing is a source, everything is information and nothing is information. On several occasions, the term infoma used by Byung Chul Han to describe those‘agents who process information‘ has been commented on. This aspect, in the age of the Internet, is further aggravated by the spread of information sources. While information sources were originally known and information could therefore be defined as “News, data or element that enables one to have more or less exact knowledge of facts, situations, ways of being.” (Source: Treccani), today we have moved from knowledge by information to knowledge by opinion, where opinion is defined as “the concept that one or more persons form about particular facts, phenomena, manifestations, when, lacking a criterion of absolute certainty to judge their nature” (Source: Treccani).
With knowledge by opinion, it is not possible to create reliable training, because the requirements that make sources ‘reliable’ are missing.
Managing training
Man has used rationality to control scientific phenomena: this is an incontrovertible fact on which the scientific disciplines are based first. It is a processthat Weber Weber describes as“that action which, based on an evaluation of its possible consequences, presents itself as the most appropriate for achieving the desired goal” (Source: Treccani). This implies that algorithmic training, which originates with a neutral objective and is not conditioned by malicious motives, will mostly be achieved but may still encounter noise phenomena dictated by disturbing elements, such as unsuitable data sources.
Nobel Prize-winning economist Daniel Kahenam wrote a book called ‘ Noise: A Flaw in Human Reasoning’ focusing on the concept of noise and distinguishing it from bias. If bias is the predictable difference in some outcomes (e.g. in answers, in judgements), error can be defined as an unpredictable (and not even intended) difference between those outcomes. But noise is present, taken for granted, part of human nature and social activities, and escaping from it is an arduous task but only possible with control processes aimed at its reduction. In order to bring about this reduction, processes that could be described as ‘decision standardisation’ have been developed, created to minimise individual influences in the cognitive process. This is demonstrated by forensic sciences, which, in order to preserve legal evidence, have created procedures aimed at reducing noise to a minimum on the basis of technical-scientific results and good practice.
Is an image a reliable source? What about a text?
When we talk about training, we are talking about an exercise process that makes use of tools, materials, data, and this raises a further problem. Is an image, the source of which one may be unaware, still a reliable source? A photograph, at least apparently, is immutable in its meaning regardless of the source that provides it, let us try an example.
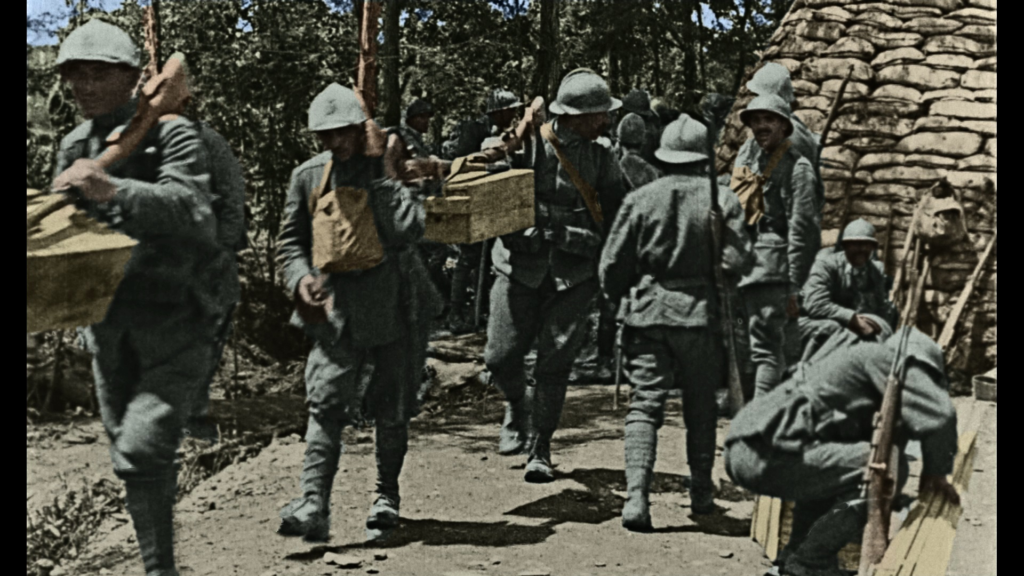
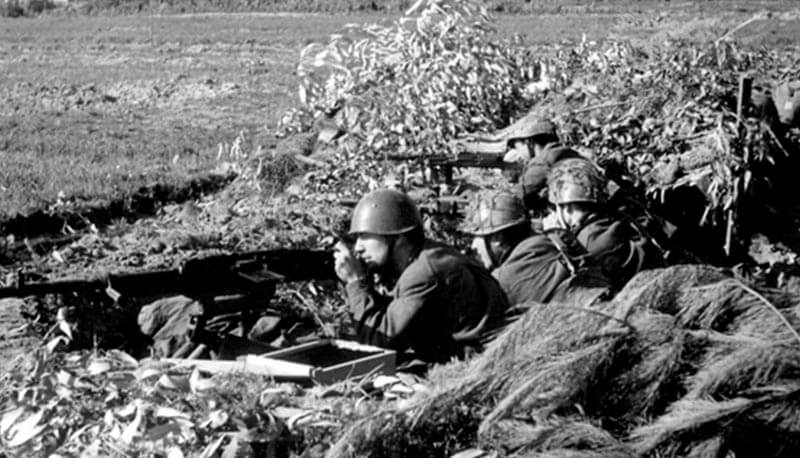
The image on the left shows a context of war, of this there is no doubt, and this is conveyed by the photograph in an absolutely unequivocal manner. The image in itself is a reliable source to portray the context, but this image is in fact artefactual as it comes from a scene of a film and has no documentary pretence but only narrative. It depicts a verisimilar but not real reality. The photo on the right also shows a wartime context but is more reliable as it is taken directly by a journalist of the time. Both could be considered reliable, both seem to tell the same scenario but only one of them is actually supposed to do so preserving the characteristic of reliability.
There are therefore two essential pieces of information in this case: the first piece of information concerns the context represented, but the second piece of information concerns the original source, which enhances the image on the right more than the one on the left. Ultimately, with the same context, one is more important than the other because it benefits from originality.
Yet the source is not immediately communicated; rather, the source must be sought and verified. Yet it is the element that makes the difference in value between the two images.
Conclusions
In the article, an attempt was made to explain how discrimination is a ‘human’ consequence of the rationalisation process and how it can take on both malicious and culpable characteristics. The attempt to limit discrimination requires processes based on acquired and verifiable good practices: whose sources are known and authoritative. Training is based on at least two subjects: the trainer and the trainee; the former must have specific skills necessary for the latter, but also the appropriate method to transmit them. Certainly, the challenge towards a transparent and effective artificial intelligence is just beginning.